How AI Trends Are Transforming Financial Services and Beyond
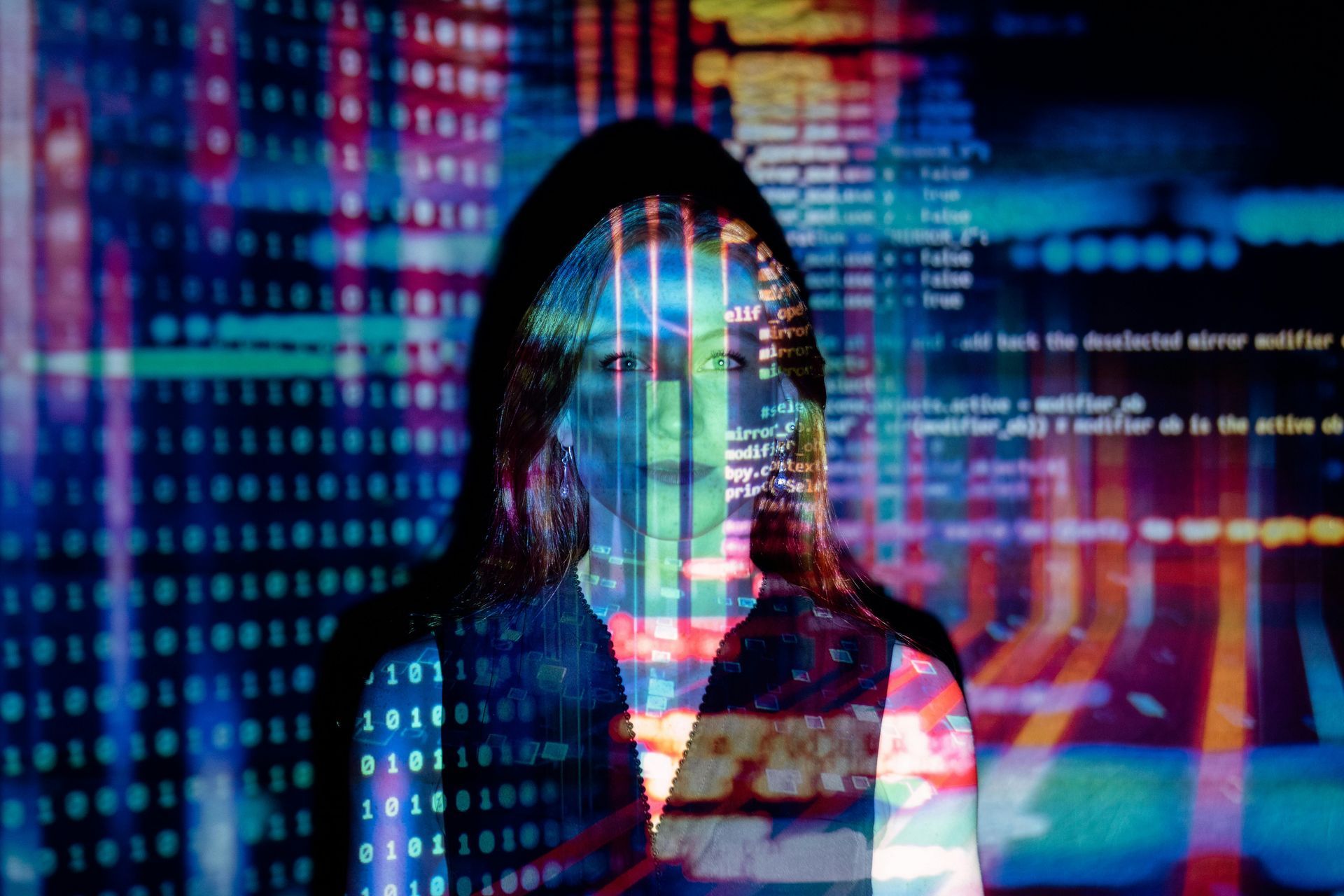
AI Trends in Action for Financial Services
This article examines transformative AI trends with a specific focus on their application in financial services. Through practical examples and insights, we discuss how businesses can harness AI to enhance efficiency, drive customer engagement, and maintain competitive advantage. A people-centric perspective is woven throughout, highlighting the importance of workforce empowerment and cultural alignment. The article also delves into AI’s evolution, offering lessons from the past and a forward-looking perspective on its future trajectory.
1. Generative AI and Large Language Models (LLMs)
Generative AI, powered by models like ChatGPT, is reshaping communication and personalization in financial services.
Example
Chatbots powered by Large Language Models (LLMs) enable banks to provide 24/7 personalized customer service. Tools like Jasper AI help compliance teams generate regulatory documentation quickly and accurately.
Strategic Implications
Automates repetitive tasks, enabling teams to focus on higher-value activities.
Challenges
Managing data security and ensuring ethical use.
- Root Cause: Insufficient governance frameworks to address data handling and algorithmic transparency.
- Solution: Develop robust policies for data privacy and bias mitigation, along with regular audits of AI systems.
People-Centric Insight
Empower employees by training them to supervise and refine generative AI outputs, fostering trust and efficiency.
2. Augmented Decision-Making with AI
AI enhances decision-making by providing actionable insights through data analysis.
Example
Fraud detection systems use machine learning to identify unusual patterns in transactions. Wealth management platforms employ predictive analytics for portfolio recommendations.
Strategic Implications
Improves accuracy, speeds up decision-making, and mitigates risks.
Challenges
Balancing AI recommendations with human oversight.
- Root Cause: Over-reliance on AI outputs without clear guidelines for human intervention.
- Solution: Establish decision frameworks that define when human judgment must override AI recommendations and provide employee training to interpret AI insights effectively.
People-Centric Insight:
Equip teams with tools and training to interpret AI insights while retaining ultimate accountability.
3. Edge AI
Edge AI enables real-time processing by analyzing data locally, without relying on cloud connectivity.
Example
Mobile payment systems use edge AI for secure and instantaneous transaction verification. Insurance companies deploy it in telematics devices to monitor driving behaviors.
Strategic Implications
Enhances operational reliability and data security.
Challenges
High initial setup costs and integration with existing systems.
- Root Cause: Limited compatibility between new AI technologies and legacy infrastructure.
- Solution: Gradually modernize IT systems while conducting pilot programs to test edge AI use cases before scaling.
People-Centric Insight
Involve employees in deploying and maintaining edge AI to ensure its seamless operation and ethical application.
4. Responsible AI and Governance
As AI adoption accelerates, governance frameworks must address ethical concerns and regulatory compliance.
Example
AI-driven credit scoring systems must comply with fairness standards. Companies like Salesforce implement bias detection tools to ensure equitable decision-making.
Strategic Implications
Builds customer trust and meets regulatory requirements.
Challenges
Ensuring transparency in "black-box" algorithms.
- Root Cause: Complexity of AI models that lack explainability.
- Solution: Invest in explainable AI technologies and create interdisciplinary teams to review and document AI decision-making processes.
People-Centric Insight
Foster a culture of accountability where employees understand and uphold ethical standards in AI use.
5. AI for Sustainability
AI supports sustainability initiatives by optimizing resource usage and reducing waste.
Example
Financial institutions use AI for ESG (Environmental, Social, and Governance) analysis, identifying green investment opportunities. AI-driven tools help banks reduce energy consumption in data centers.
Strategic Implications
Positions organizations as leaders in sustainability while improving efficiency.
Challenges
Aligning sustainability goals with business priorities.
- Root Cause: Lack of alignment between sustainability metrics and operational KPIs.
- Solution: Develop cross-functional teams to integrate ESG goals into AI project roadmaps and track progress through unified performance dashboards.
People-Centric Insight:
Engage employees in sustainability efforts by demonstrating how AI-driven initiatives benefit both the environment and the organization.
The Role of People in AI Adoption
AI’s success hinges on a people-centric approach. Here’s why it matters:
Cultural Alignment | AI adoption requires a culture that embraces change and fosters innovation. | |
Upskilling and Empowerment | Empower employees with the skills needed to leverage AI effectively, ensuring confidence and competence. | |
Human Oversight | Maintain accountability by positioning AI as a tool to support, not replace, human decision-making. | |
Customer Connection | Use AI to enhance personalized customer experiences while retaining the human touch that builds trust and loyalty. |
Historical Context: Lessons for Financial Services
AI’s evolution offers valuable lessons for financial services:
- 1950s-1980s: Early AI systems, like rule-based algorithms, laid the groundwork for today’s advancements.
- 2000s-Present: AI matured into a general-purpose technology, enabling applications such as automated trading and fraud detection.
Takeaway: The financial sector’s cautious yet proactive approach to adopting emerging technologies highlights the importance of balancing innovation with reliability.
Future Outlook: Prioritizing Technology and People
As AI evolves, financial services must focus on:
- Iterative Transformation: Foster a culture of experimentation and learning to adapt quickly to technological advances.
- Human-AI Collaboration: Position AI as an enabler, not a replacement, of human expertise.
- Ethical Practices: Prioritize transparency, inclusivity, and regulatory alignment to maintain trust.
Conclusion
AI is a powerful enabler of transformation in financial services, driving innovation, improving efficiency, and enhancing customer experiences. However, success depends on more than just adopting cutting-edge technologies—it requires a people-centric approach that aligns with organizational values and customer expectations.
How Leaders Can Apply This Information
Strategic Implementation
Use the insights to identify high-impact areas for AI, such as fraud detection, compliance automation, and customer personalization.
Empowering the Workforce
Develop training programs to upskill employees in AI tools and foster a culture that embraces innovation.
Governance and Ethics
Establish frameworks that ensure ethical AI use, align with regulatory requirements, and maintain customer trust.
Iterative Piloting
Begin with small-scale AI initiatives to demonstrate value and refine processes before scaling.
Sustainability Integration
Leverage AI to meet ESG goals, strengthening both operational efficiency and environmental responsibility.
Advised Next Steps
- Conduct an organizational readiness audit to evaluate AI capabilities.
- Launch pilot programs focused on quick wins, such as AI-powered chatbots or predictive analytics in underwriting.
- Foster collaboration between teams by embedding AI into cross-functional strategies.
- Communicate a clear vision for AI adoption, emphasizing its role in enhancing, not replacing, human expertise.
- By addressing root causes of challenges and prioritizing these actions, leaders can unlock AI’s full potential to drive sustainable growth, foster trust, and deliver lasting value in financial services.